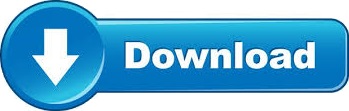
The errors, an ARIMA model it is NOT a linear regression model, because there

Period (LAG(Y,1) in Statgraphics or Y_LAG1 in RegressIt). Regression model in which the independent variable is just Y lagged by one Regression model and which could be fitted with standard regressionįirst-order autoregressive (“AR(1)”) model for Y is a simple (“self-regressed”) model, which is just a special case of a Predictors consist only of lagged values of Y, it is a pure autoregressive Recent values of Y and/or a weighted sum of one or more recent values of Predicted value of Y = a constant and/or a weighted sum of one or more
ARIMA IN ASREML SERIES
Signal is then extrapolated into the future to obtain forecasts.įorecasting equation for a stationary time series is a linear (i.e., regression-type) equation in which the predictors “filter” that tries to separate the signal from the noise, and the Sign, and it could also have a seasonal component. Of signal and noise, and the signal (if one is apparent) could be a pattern ofįast or slow mean reversion, or sinusoidal oscillation, or rapid alternation in A random variable of this form can be viewed (as usual) as a combination (correlations with its own prior deviations from the mean) remain constant over The latter condition means that its autocorrelations Its variations around its mean have a constant amplitude, and it wiggles in aĬonsistent fashion, i.e., its short-term random time patterns always look Random variable that is a time series is stationary if its statistical With nonlinear transformations such as logging or deflating (if necessary). “stationary” by differencing (if necessary), perhaps in conjunction Models for forecasting a time series which can be made to be
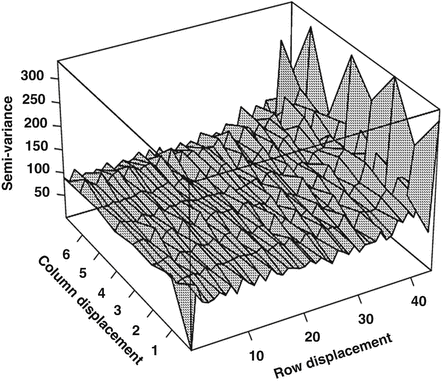
Forecasting equation: ARIMA models are, in theory, the most general class of
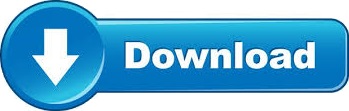